Article• 3 minute read
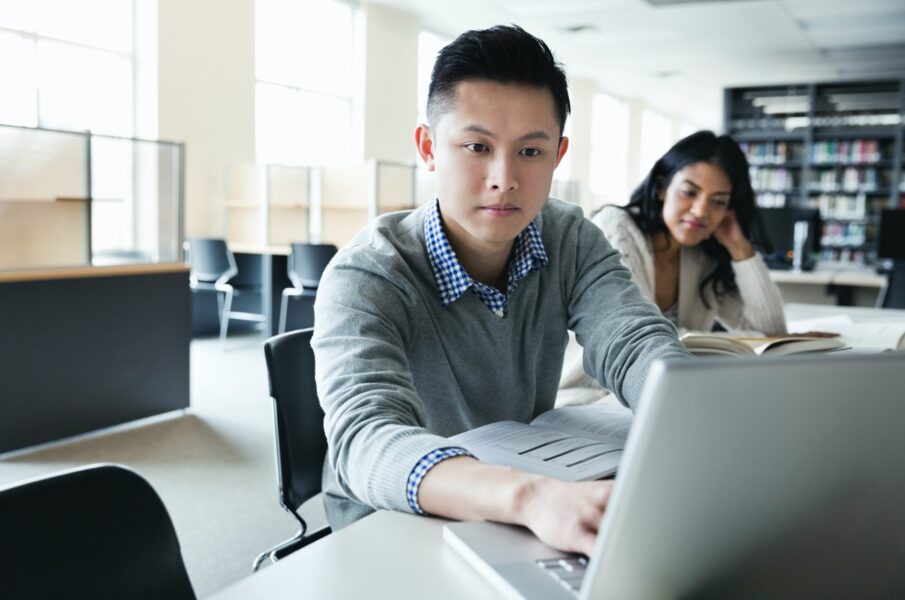
Natural Language Processing (NLP), a subfield of Artificial Intelligence (AI), is reshaping the landscape of medical education assessment by offering innovative avenues to evaluate essential skills. In collaboration with fellow scientists, my team conducts extensive research to develop NLP and AI capabilities tailored to the unique needs of the field. This post highlights the core tenets of our approach to innovation: driving research excellence and promoting transparency through collaboration and active community engagement.
AI Capabilities Tailored to the Needs of Medical Education Assessment
To evaluate complex constructs like communication and clinical reasoning, medical educators require innovative assessments that go beyond the multiple-choice format. However, such assessments require the ability to efficiently score a vast number of free-text responses or deliver personalized feedback to large learner groups within tight timelines. My team specializes in developing NLP/AI capabilities, including technology-assisted scoring, improved content creation, and automated provision of personalized feedback. Recent applications include a system for scoring responses to open-ended items, predicting item difficulty based on text, and facilitating clinical item writing through automated distractor suggestion.
Progress and Transparency Through Community Engagement
In our pursuit of developing specialized AI solutions, we recognize the dual imperative for rapid progress and responsible, transparent AI research practices. To navigate this balance, we lean into community engagement by openly sharing data and extending invitations for NLP/AI researchers to contribute in creating open-access solutions available for public scrutiny and evaluation. We also bring together researchers across AI, assessment, and medical education to form a shared understanding of key challenges. Examples of such initiatives include:
- The BEA’24 Shared Task on Automated Prediction of Item Difficulty and Item Response Time. In this research competition, we’ve challenged researchers to develop innovative ways for automated prediction of item difficulty and response time — a key requirement for automated generation of test items at desired difficulty levels. The approaches developed by the competing teams will be published in an open-access form in the Association of Computational Linguistics’ (ACL) Anthology.
- NBME’s Kaggle competition on Automated Scoring of Clinical Patient Notes. This competition tackled the most significant barriers in the automated scoring of OSCE assessments. Approximately 1500 teams from around the world competed to score patient notes from the former USMLE Step 2 Clinical Skills exam. The full code of all winning solutions is available on the competition’s website.
- Natural Language Processing in Assessment, an NBME Educational Virtual Conference and the resulting NCME book series volume Advancing Natural Language Processing in Educational Assessment. The conference provided a forum for researchers from the NLP, assessment, and medical education fields to discuss new ways to use NLP/AI to improve assessment. The result of the conference learnings was a first-of-its-kind book that provides evidence-based practices for the use of NLP in assessment.
By fostering community engagement in AI for medical education, we uphold transparency and excellence in research, while cultivating a diverse group of researchers to propel advancements in critical areas. As an institution that values partnership, we share innovations publicly, allowing other organizations to build upon these solutions. Together, we are shaping the future of AI in medical education assessment.